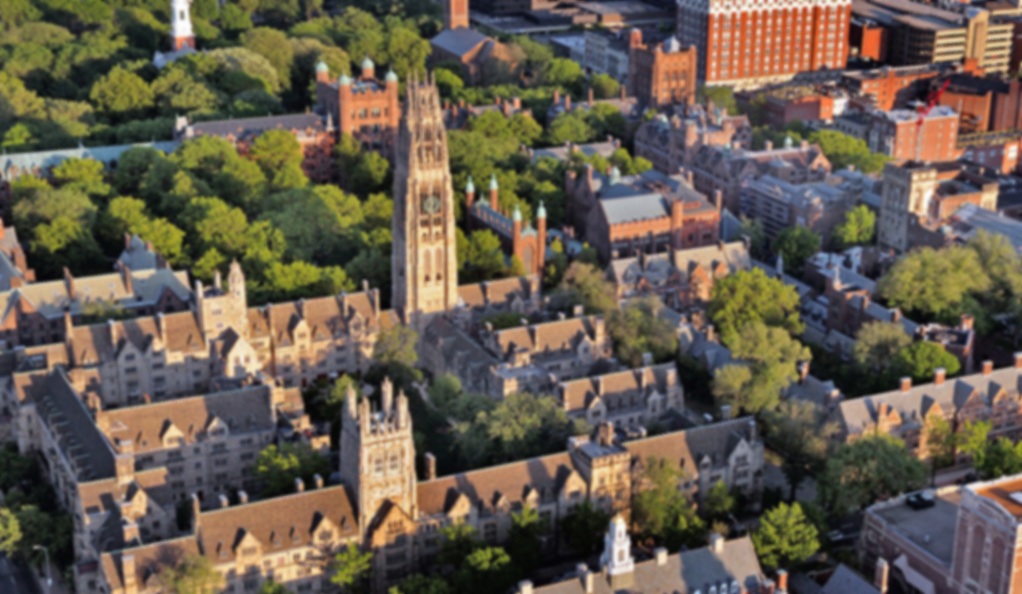
News
Our Research
At the van Dijk Lab we advance artificial intelligence and large‑scale foundation models for biomedicine, blending rigorous mathematics, state‑of‑the‑art ML, and rich genomic and clinical data to uncover the rules of life.
Multiscale Foundation Models
We engineer large‑scale foundation models that learn across biological scales—from single molecules to whole organs. By casting omics data as a biological language, our models construct virtual cells that decode cellular programs driving cancer, autoimmune disease, and tissue regeneration. At the organ level, we fuse neuroimaging, electrophysiology, cardiac MRI, ECG, and genomics to build digital twins that forecast disease progression and therapeutic response.
Spatiotemporal Dynamical Systems & Neural Operator Learning
Biological processes are inherently dynamical. We recast them as high‑dimensional partial‑differential or integral equations and learn the governing operators directly from data. Continuous‑time transformers, graph neural operators, and integral‑equation networks developed in the lab capture long‑range, history‑dependent interactions, enabling in‑silico experiments that model brain‑activity dynamics from functional MRI, cardiac electromechanical and flow dynamics from MRI and ECG, and fluid dynamics governed by Navier–Stokes equations.
Causality, Counterfactuals, and Interventions
Moving beyond correlation, our algorithms map causal structure and predict the impact of interventions. We deploy large language models as causal reasoners that infer cellular responses to gene edits or drug perturbations, forecast clinical‑trial outcomes, and enable virtual personalized trials where clinicians can compare therapies in silico.
Theory of Intelligence
Our curiosity reaches further to the foundations of artificial intelligence itself. By studying learning in critical dynamical regimes—the so‑called edge of chaos—we uncover principles that make reasoning efficient, robust, and adaptable. These insights inform new architectures that balance expressive power with interpretability.
Selected Projects
Cell2Sentence
Cell2Sentence bridges transcriptomics and NLP by encoding scRNA‑seq profiles as “cell sentences” to fine‑tune LLMs for tasks like cell generation and annotation. ICML 2024.
C2S‑Scale scales this framework to 27 billion parameters trained on a billion‑token multimodal corpus—achieving state‑of‑the‑art predictive and generative performance for complex, multicellular analyses. bioRxiv 2025 (Preprint).
CINEMA-OT
CINEMA‑OT applies causal inference with optimal transport to disentangle true treatment effects from confounders in single‑cell perturbation experiments, outperforming existing methods on both simulated and real data. It uncovers mechanisms behind impaired antiviral responses in airway organoids and chemokine‑driven immune recruitment in cytokine‑stimulated immune cells. Nature Methods 2023
Intelligence at the Edge of Chaos
By training LLMs on elementary cellular automata rules of varying complexity, we pinpoint a “sweet spot” of data complexity that maximizes downstream predictive and reasoning abilities. Our findings suggest that exposing models to appropriately complex patterns is key to unlocking emergent intelligence. ICLR 2025.
MAGIC
MAGIC leverages Markov affinity–based graph diffusion to impute missing transcripts in single‑cell RNA‑seq data, effectively denoising dropout noise and restoring gene–gene relationships. In epithelial‑to‑mesenchymal transition analyses, MAGIC reveals a continuous spectrum of intermediate, stem‑like cell states and uncovers both established and novel regulatory interactions. Cell 2018
Selected publications:
Rizvi, et al. Scaling Large Language Models for Next-Generation Single-Cell Analysis. bioRxiv, 2025.
Caro, et al. BrainLM: A Foundation Model for Brain Activity Recordings. ICLR 2024.
Levine, et al. Cell2Sentence: Teaching Large Language Models the Language of Biology. ICML 2024.
Zappala, et al. Learning integral operators via neural integral equations. Nature Machine Intelligence 2024.
Dong, et al. Causal identification of single-cell experimental perturbation effects with CINEMA-OT. Nature Methods, 2023.
van Dijk, et al. Recovering Gene Interactions from Single-Cell Data Using Data Diffusion Cell 2018.
GitHub
Discover our latest projects and code on GitHub and HuggingFace!
Revolutionizing Biomedical Research with Machine Learning
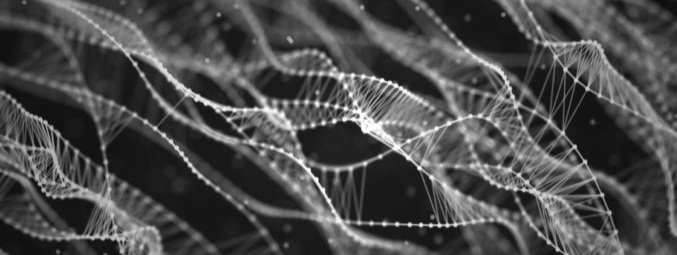
Join Our Team: Shape the Future of Biomedicine with Machine Learning
Who We're Looking For
Are you passionate about leveraging machine learning to drive groundbreaking advancements in biology and medicine? The Van Dijk Lab is actively seeking talented individuals to join our interdisciplinary team. We have openings for interns, students, postdocs, programmers, and staff researchers.
Preferred Qualifications
While a background in Computer Science, Mathematics, or Engineering is preferred, no prior experience in biology is required. What's essential is your enthusiasm for working with real-world data and your interest in either crafting innovative algorithms or applying them to solve complex problems.
Why Join Us?
As part of both Yale Internal Medicine and Computer Science departments, we are uniquely positioned at the intersection of computational and biomedical research. Located at the Yale Medical School, our lab collaborates closely with clinicians, granting us access to some of the most compelling datasets in the field.
Our Impact
Our dual focus allows us to make significant contributions to both biomedicine—publishing in top-tier biological and medical journals—and computer science—presenting our findings at leading CS and ML conferences.
How to Apply
For more information or to express your interest, please reach out to Dr. David van Dijk at david.vandijk (at) yale.edu.
Excited about Machine Learning and Biomedicine?
Join our Lab
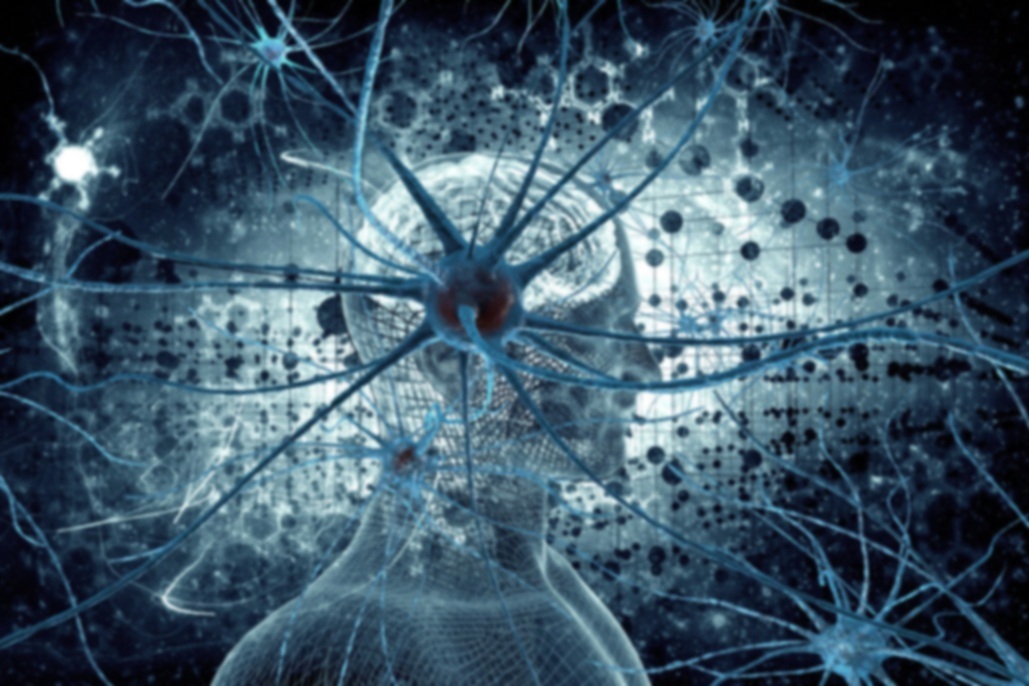